
Bias should not be confused with chance variability that causes some findings to be false by chance even though the study design, data, analysis, and presentation are perfect. Let u be the proportion of probed analyses that would not have been “research findings,” but nevertheless end up presented and reported as such, because of bias. Since usually the vast majority of investigators depend on a = 0.05, this means that a research finding is more likely true than false if (1 - β) R > 0.05.įirst, let us define bias as the combination of various design, data, analysis, and presentation factors that tend to produce research findings when they should not be produced. A research finding is thus more likely true than false if (1 - β) R > α. According to the 2 × 2 table, one gets PPV = (1 - β) R/( R - βR + α). have called the false positive report probability. The PPV is also the complementary probability of what Wacholder et al. After a research finding has been claimed based on achieving formal statistical significance, the post-study probability that it is true is the positive predictive value, PPV.
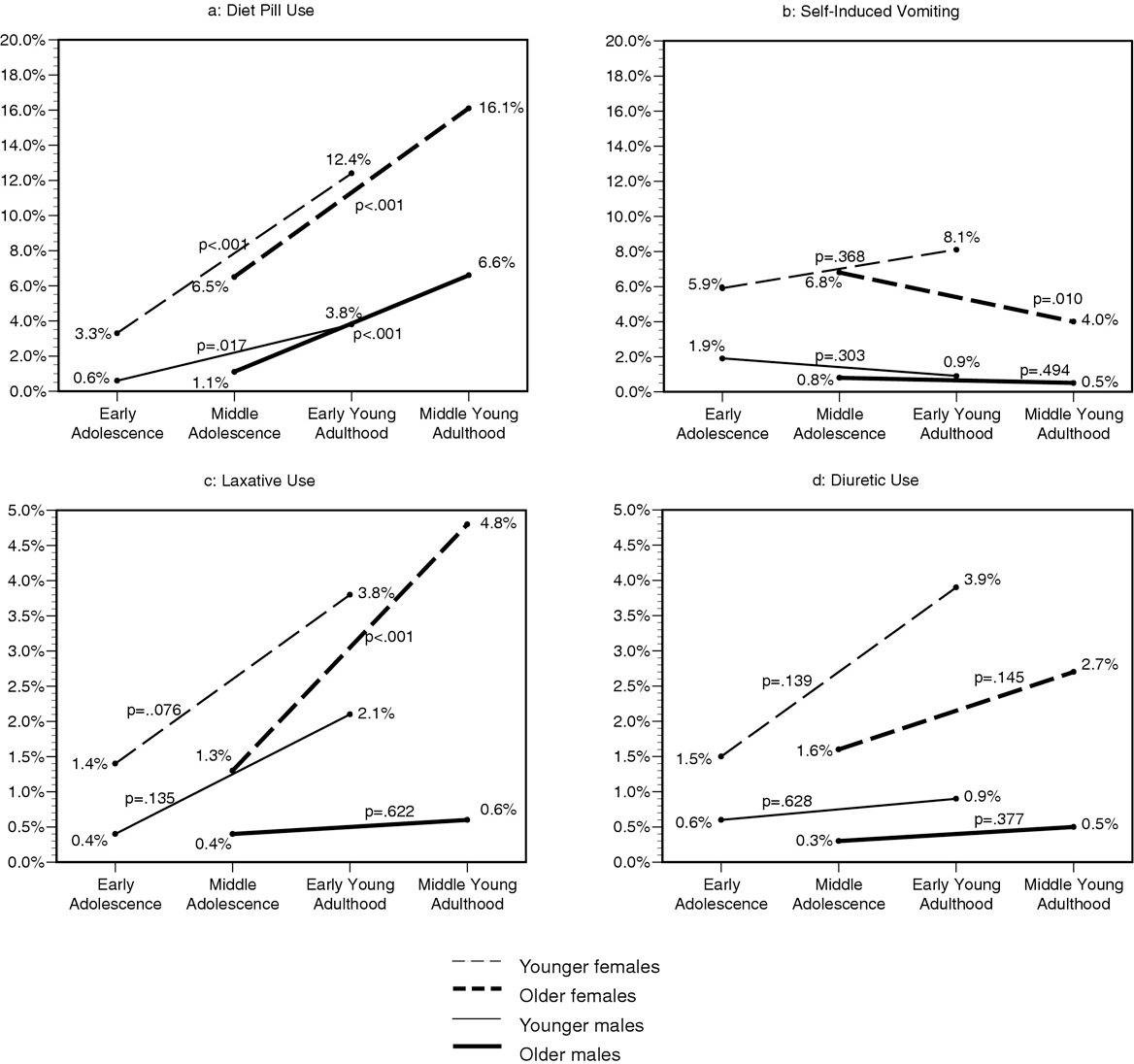
Assuming that c relationships are being probed in the field, the expected values of the 2 × 2 table are given in Table 1. The probability of claiming a relationship when none truly exists reflects the Type I error rate, α. The probability of a study finding a true relationship reflects the power 1 - β (one minus the Type II error rate). The pre-study probability of a relationship being true is R/( R + 1). Let us also consider, for computational simplicity, circumscribed fields where either there is only one true relationship (among many that can be hypothesized) or the power is similar to find any of the several existing true relationships. R is characteristic of the field and can vary a lot depending on whether the field targets highly likely relationships or searches for only one or a few true relationships among thousands and millions of hypotheses that may be postulated. Let R be the ratio of the number of “true relationships” to “no relationships” among those tested in the field. In a research field both true and false hypotheses can be made about the presence of relationships. Consider a 2 × 2 table in which research findings are compared against the gold standard of true relationships in a scientific field. It can be proven that most claimed research findings are falseĪs has been shown previously, the probability that a research finding is indeed true depends on the prior probability of it being true (before doing the study), the statistical power of the study, and the level of statistical significance. However, here we will target relationships that investigators claim exist, rather than null findings. “Negative” is actually a misnomer, and the misinterpretation is widespread. Research findings are defined here as any relationship reaching formal statistical significance, e.g., effective interventions, informative predictors, risk factors, or associations. Research is not most appropriately represented and summarized by p-values, but, unfortunately, there is a widespread notion that medical research articles should be interpreted based only on p-values. Several methodologists have pointed out that the high rate of nonreplication (lack of confirmation) of research discoveries is a consequence of the convenient, yet ill-founded strategy of claiming conclusive research findings solely on the basis of a single study assessed by formal statistical significance, typically for a p-value less than 0.05. Modeling the Framework for False Positive Findings In this essay, I discuss the implications of these problems for the conduct and interpretation of research. Moreover, for many current scientific fields, claimed research findings may often be simply accurate measures of the prevailing bias. Simulations show that for most study designs and settings, it is more likely for a research claim to be false than true. In this framework, a research finding is less likely to be true when the studies conducted in a field are smaller when effect sizes are smaller when there is a greater number and lesser preselection of tested relationships where there is greater flexibility in designs, definitions, outcomes, and analytical modes when there is greater financial and other interest and prejudice and when more teams are involved in a scientific field in chase of statistical significance. The probability that a research claim is true may depend on study power and bias, the number of other studies on the same question, and, importantly, the ratio of true to no relationships among the relationships probed in each scientific field.
There is increasing concern that most current published research findings are false.
